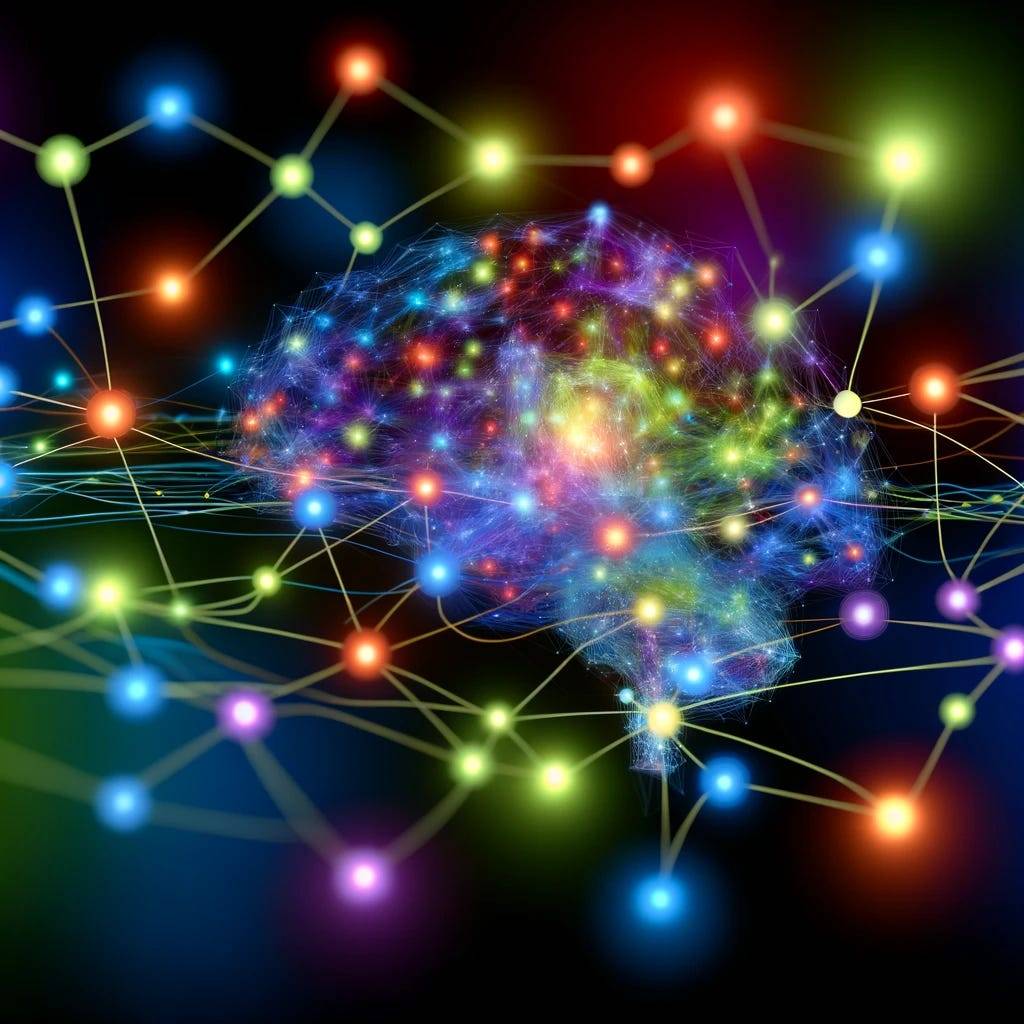
Imagine if we could predict outcomes before they happen, like forecasting a storm or even guessing the winner of a soccer game. In the world of machine learning, scientists create models that make these predictions possible. One fascinating aspect of this is the use of something called “surrogate losses.” These are special functions that help us train a model when the actual prediction task might be too difficult to tackle directly.
The Unsung Heroes
Surrogate losses are a bit like training wheels for a bicycle — they make the learning process smoother and manageable. In binary and multi-class classification, which are ways to categorize data into two or more classes, surrogate losses help by simplifying complex relationships in data. This simplification allows computers to learn quickly and effectively, making accurate predictions based on past data.
A World of Possibilities
As we dive deeper into the realm of machine learning, we encounter multi-class classification. This method is used in more complex scenarios where decisions aren’t just binary. For instance, instead of deciding if an image is of a cat or a dog, we might also consider lions, tigers, and bears! Surrogate losses help manage this complexity by providing a framework to handle multiple categories smoothly.
Growth Rates and Learning Efficiency
An important discovery in the use of surrogate losses is their growth rate in learning algorithms. This growth rate determines how quickly a learning model can improve its accuracy. Recent studies have shown that certain types of surrogate losses have a universal “square-root” growth rate. This means they provide a consistent way to measure and predict how improvements in learning methods translate to better performance in practical tasks.
Surrogate Losses in Neural Networks
In neural networks, which are complex models inspired by the human brain, surrogate losses are particularly powerful. They allow these networks to learn from vast amounts of data without getting overwhelmed by the complexity. This ability is crucial for tasks like voice recognition or translating languages, where the nuances of human communication must be captured.
Here’s a graph below that helps us understand how surrogate losses consistently support learning across various models, with notable improvements in prediction accuracy, especially in complex applications like neural networks.:
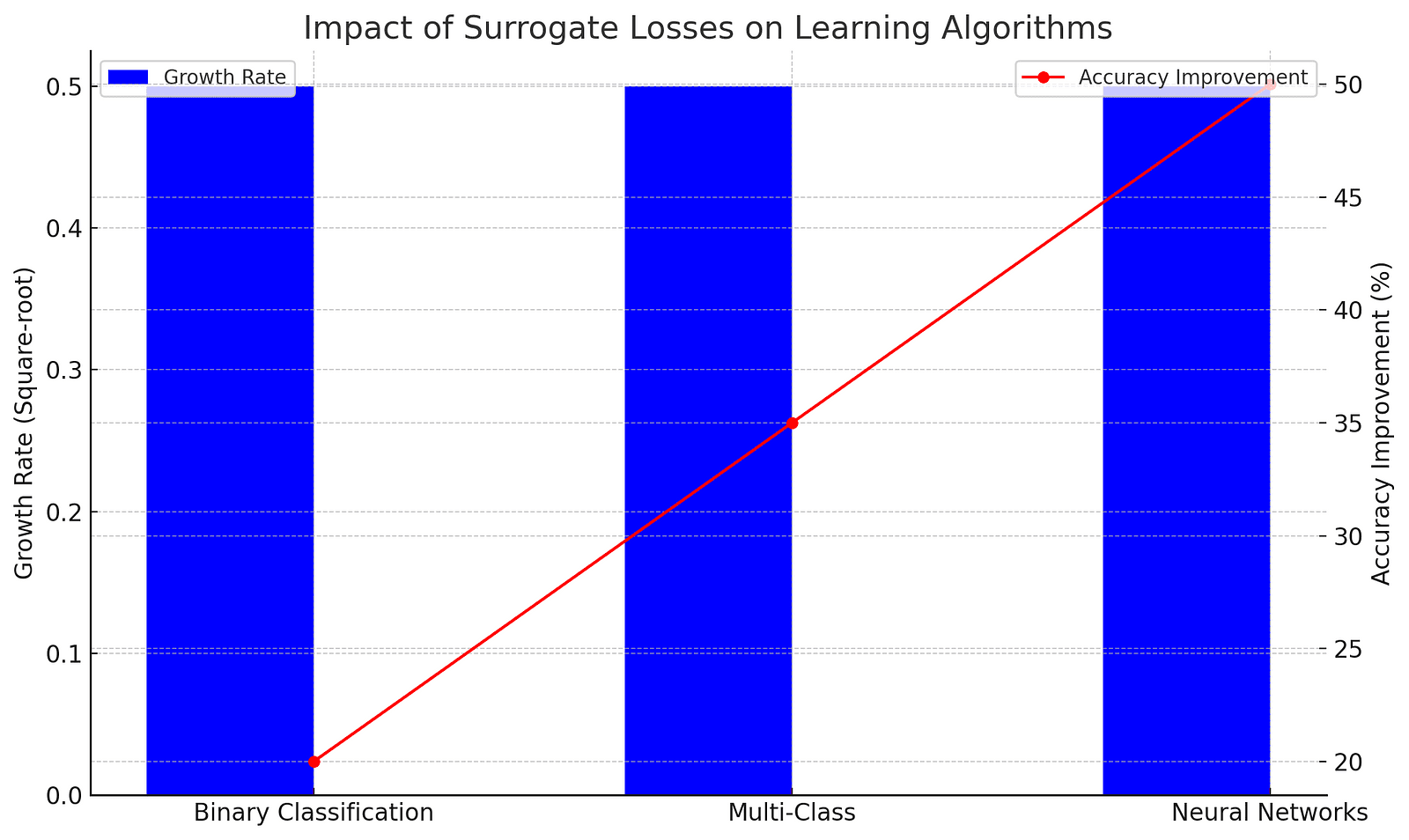
Universal Applicability
Surrogate losses are not limited to one type of learning model; they are universally applicable across various machine learning algorithms. This universality makes them incredibly valuable in the field of artificial intelligence.
Enhancement of Learning Speed
By simplifying the original learning problem, surrogate losses can significantly speed up the learning process. This acceleration is vital for applications that require real-time decision-making, such as autonomous driving.
Improved Prediction Accuracy
When surrogate losses are optimized, they lead to improved prediction accuracy. This improvement is because these losses help in fine-tuning the parameters of the learning models more effectively.
Handling of Non-Differentiable Problems
Many real-world problems are non-differentiable, meaning they can’t be solved with traditional mathematical tools. Surrogate losses enable learning algorithms to handle these problems by approximating them in a differentiable way.
Aiding Complex Classifications
In multi-class classification scenarios, surrogate losses help manage the complexity by breaking down the problem into simpler steps. This method ensures that the learning algorithm remains efficient and effective, even as the complexity of the tasks increases.
A Bright Future with Surrogate Losses
The journey into the world of surrogate losses opens up a landscape filled with potential and promise. These tools not only enhance the capability of learning algorithms but also pave the way for innovations that could transform our daily lives. From smarter healthcare diagnostics to more intuitive technology interfaces, the possibilities are endless. As we continue to explore and refine these methods, we can look forward to a future where technology understands and assists us in increasingly sophisticated ways, making our lives easier and more connected.
About Disruptive Concepts
https://www.disruptive-concepts.com/
Welcome to @Disruptive Concepts — your crystal ball into the future of technology. 🚀 Subscribe for new insight videos every Saturday!