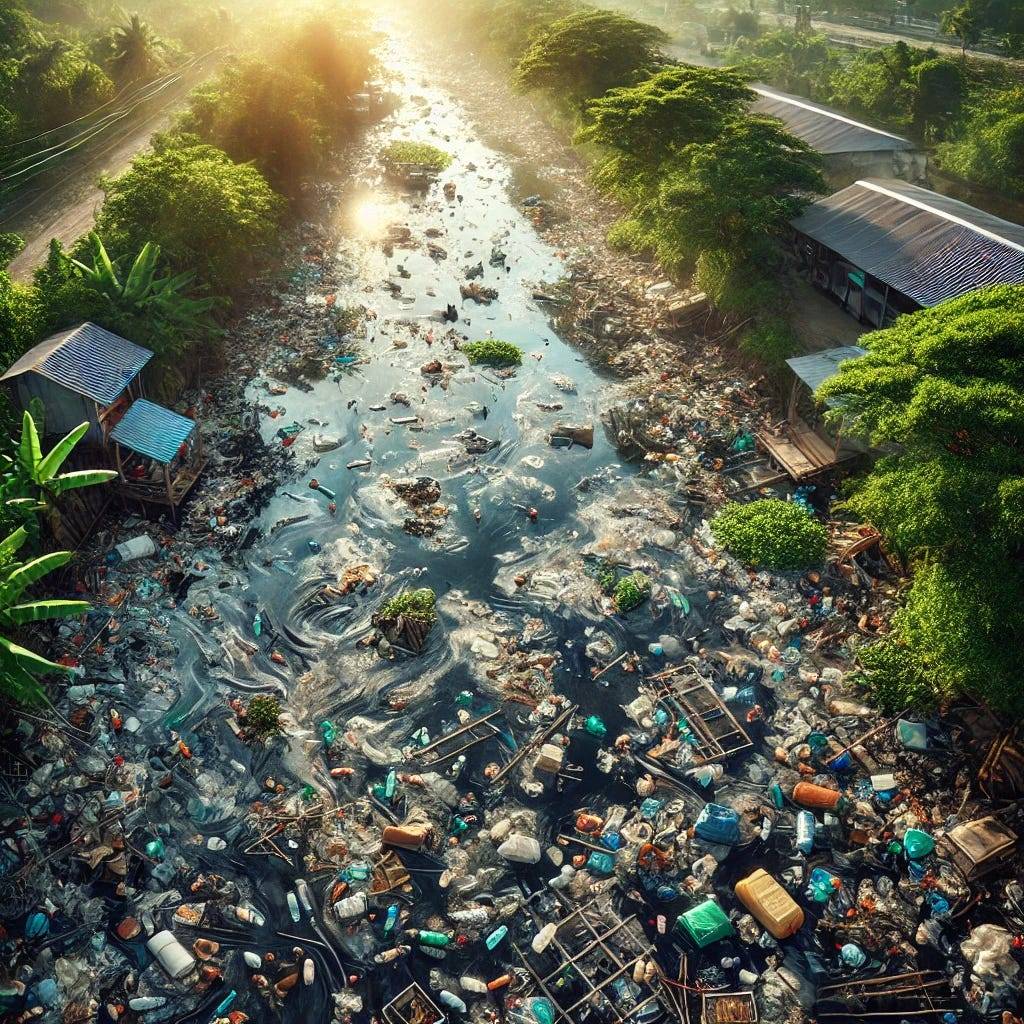
In a world where pollution is silently choking rivers, technology is rising to the challenge. The Riverine Patch Trash Segmentation (RIPTSeg) dataset — a collection of high-resolution images depicting trash-strewn rivers — is the new frontier. Here, artificial intelligence models compete to identify and segment patches of trash, a task crucial for effective cleanup. The showdown is between foundation models (FMs) and finetuned models. Surprisingly, the nimble YOLOv8 model, trained with just 30 images, outperformed its larger, more data-hungry rivals. It’s a stark reminder that in the world of AI, bigger isn’t always better. This finding could revolutionize how we approach complex environmental problems using technology.
The Rise of Finetuned Models
Foundation models, like SegGPT and PerSAM, have been hailed for their versatility across tasks. Yet, when faced with the specific challenge of identifying trash in rivers, their performance was overshadowed by a smaller, more focused model. The finetuned YOLOv8, trained on a limited but targeted dataset, showed that specialization often trumps generalization. This model’s precision in trash detection, even when data was scarce, suggests a shift in how we approach AI deployment in environmental tasks. It also hints at the untapped potential of smaller models that can be adapted quickly to new problems with minimal data.
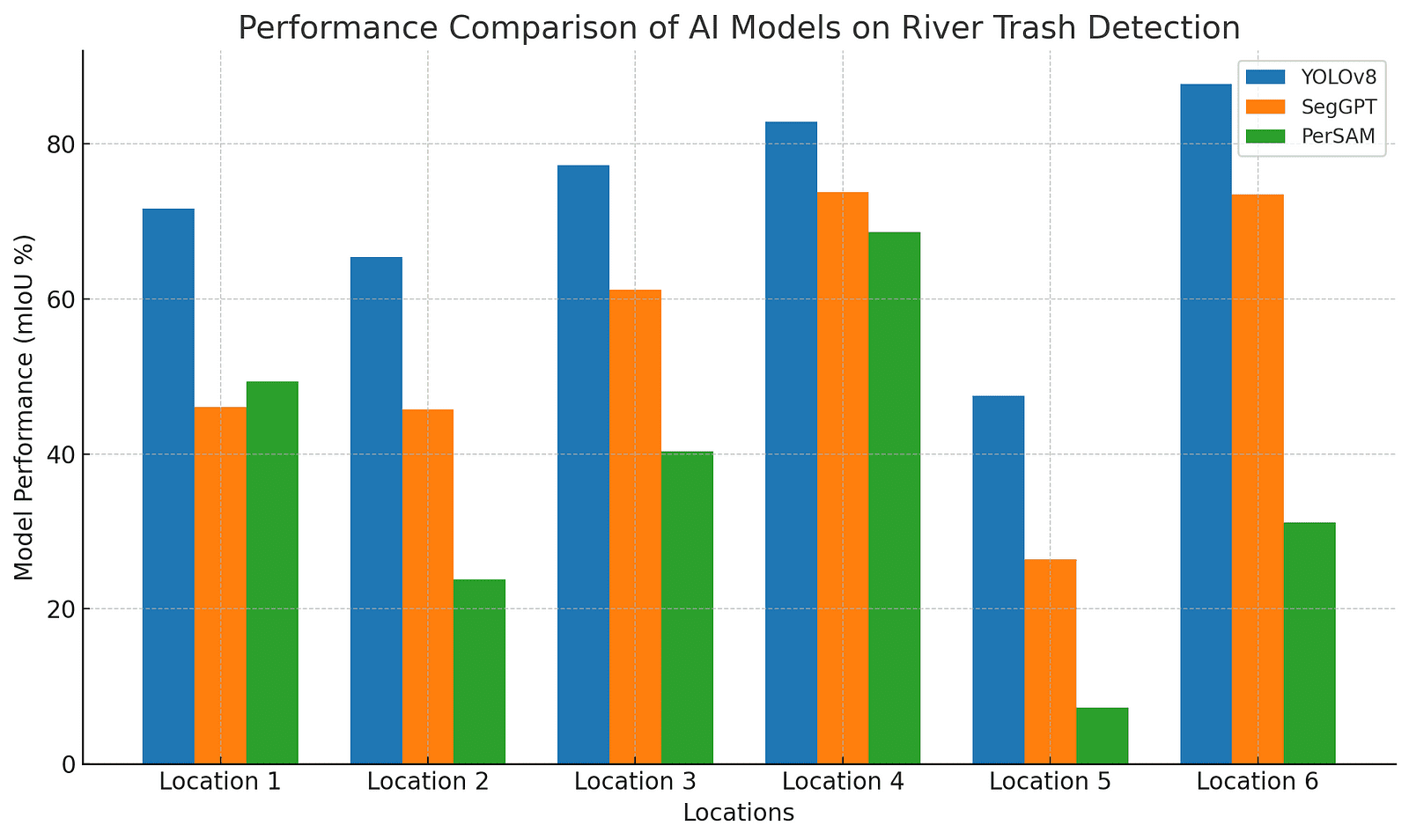
To illustrate the relative effectiveness of various AI models in detecting river pollution, we’ve prepared a visual comparison above showing how each model performed across different locations. This graph highlights the surprising success of the smaller, more specialized YOLOv8 model.
The Future of Clean Rivers
Imagine a world where rivers are no longer suffocating under heaps of plastic and debris. Thanks to AI, that future is closer than we think. With models like YOLOv8 demonstrating exceptional accuracy in detecting river trash with just a few images, the potential for real-world impact is immense. These findings could empower communities and organizations like The Ocean Cleanup to deploy AI solutions swiftly and effectively. By focusing on specific problems and leveraging technology in novel ways, we might just turn the tide on river pollution, one segmented image at a time.
Beyond Riverbanks — The Broader Implications
The implications of this research stretch far beyond riverbanks. It challenges the prevailing notion that bigger, more generalized models are always the best choice for every task. In reality, fine-tuned models can often outperform, especially when trained on datasets that are closely aligned with the problem at hand. This insight opens up a whole new world of possibilities for AI in specialized applications, from healthcare to climate science. The real lesson here is about adaptability and the importance of tailoring technology to meet the specific needs of each challenge.
Small Model, Big Impact
Despite being smaller and requiring less data, the YOLOv8 model outperformed its larger competitors in detecting river trash. This could mean quicker deployments and fewer resources needed, changing how we think about AI efficiency in real-world applications.
A New Dataset for a New Challenge
The RIPTSeg dataset is unique because it includes images of river trash that haven’t been used in AI training before. This ensures that any model trained on this data is truly learning to handle real-world pollution scenarios, making the findings more applicable to actual cleanup efforts.
Foundation Models Aren’t Always Best
While foundation models like SegGPT are great for general tasks, they didn’t perform as well on the highly specialized task of river trash detection. This discovery suggests that for specific challenges, smaller, fine-tuned models may often be the smarter choice.
Trash Beyond the Riverbanks
In some locations, river trash can be harder to detect due to natural obstructions like rocks or overgrown vegetation. Models trained on site-specific data, like those used in the study, are crucial for developing targeted cleanup strategies that consider local conditions.
AI’s Role in Environmental Rescue
By using AI to monitor and manage river pollution, we could significantly reduce the flow of plastics and waste into our oceans. This study underscores the potential of AI not just as a tool for data analysis, but as an active participant in environmental conservation.
A New Hope for Our Rivers
Our rivers are at a critical juncture. While the problems they face are complex, technology offers a new way forward. The success of fine-tuned models like YOLOv8 in detecting and managing river pollution suggests that the future of environmental cleanup may lie in the smart application of AI. As we continue to refine these tools and adapt them to the unique challenges of our planet, the dream of clean, trash-free rivers is no longer a distant hope, but a very real possibility.
About Disruptive Concepts
https://disruptive-concepts.com/
Welcome to @Disruptive Concepts — your crystal ball into the future of technology. 🚀 Subscribe for new insight videos every Saturday!
Discover the Must-Have Kitchen Gadgets of 2024! From ZeroWater Filters to Glass Containers, Upgrade Your Home with Essential Tools for Safety and Sustainability. Click Here to Transform Your Kitchen Today!