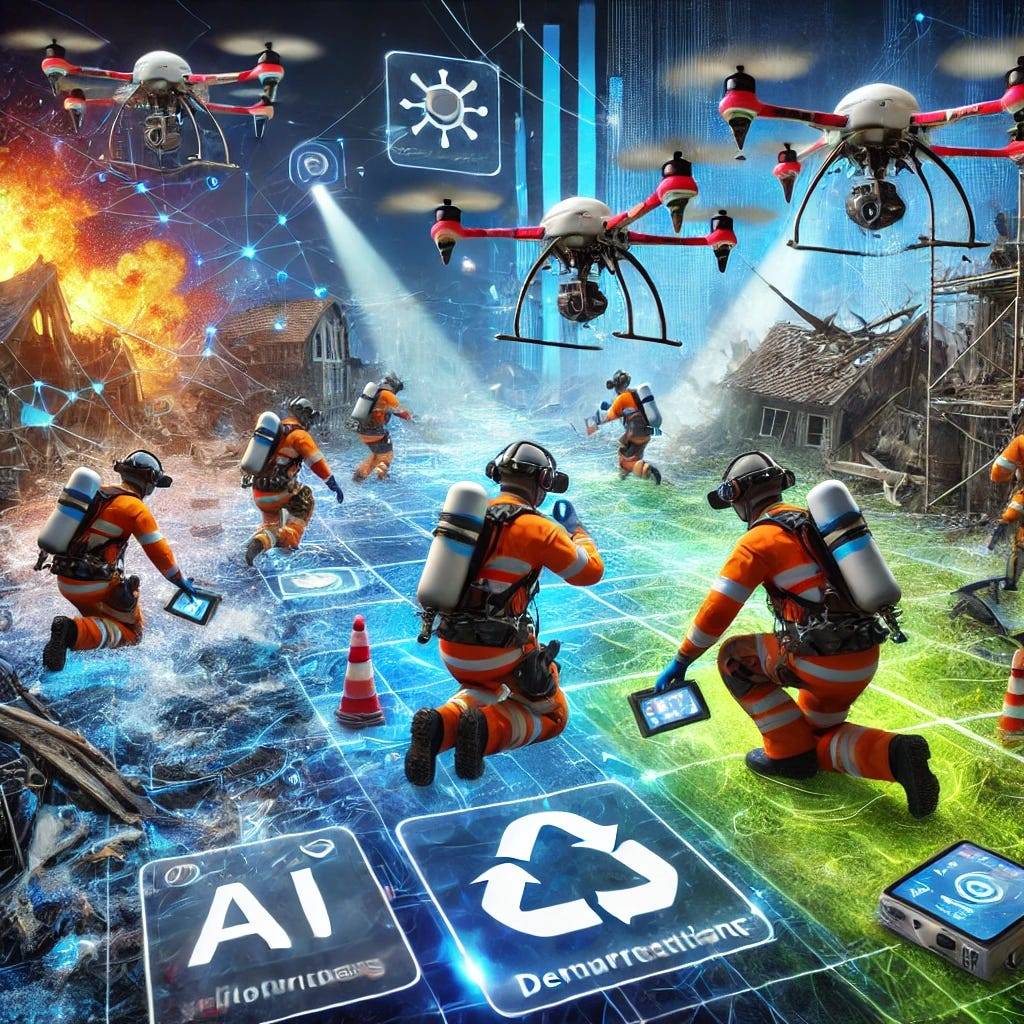
For years, we’ve taught machines to see with flat, two-dimensional images, making them pretty good at recognizing faces, reading signs, or even telling a cat from a dog. But the world isn’t flat; it’s a dynamic three-dimensional playground where everything moves, spins, and changes over time. Lexicon3D steps boldly into this challenge, redefining how machines interpret complex 3D scenes. While traditional models have focused on flat, two-dimensional images, Lexicon3D takes the leap into the dynamic depths of 3D space. By probing a range of visual foundation models — spanning from images to videos to intricate 3D point clouds — Lexicon3D reveals a new way forward. Instead of just looking at a picture and guessing what might be happening, machines can now navigate through space, interact with objects, and make decisions as if they were one of us. With 3D understanding, machines become more like real explorers, capable of operating in environments like driverless cars maneuvering through traffic or robots assisting in hospitals.
Decoding the New Language of 3D Vision
To make sense of 3D environments, scientists are creating a new kind of language for machines. It’s not about words or sentences, but about how different types of data — from images, videos, and even point clouds — are combined to make sense of our world. Think of it like a huge game of connect-the-dots, except the dots are tiny pieces of visual information scattered across time and space. By using cutting-edge models like DINOv2 and Stable Video Diffusion, machines learn to piece together these dots into meaningful pictures. These models are trained not just to recognize what they see but to understand the relationships and the geometry of the world around them.
From Pixels to Reality
Imagine standing in a room, looking around, and knowing exactly where everything is without having to walk over and touch it. That’s what 3D scene understanding enables for machines. With powerful tools like segmentation and registration, models can distinguish between different objects and align them perfectly, even when they’re partially hidden or seen from different angles. Imagine the potential: robotic arms in factories that can instantly identify faulty products or AI systems that help rescue teams navigate through disaster sites by generating 3D maps in real-time.
Breaking the Mold
There’s an interesting twist in this story: models trained to understand language aren’t always the best at handling vision tasks, especially in 3D. You’d think that combining language with visual information would make machines smarter, but it turns out that models like CLIP struggle with certain 3D tasks. This unexpected finding opens up new questions about how we train models and whether combining different types of data is always the best approach. It’s like learning that mixing all your favorite foods together doesn’t always result in a delicious meal. Sometimes, keeping things separate makes them stronger.
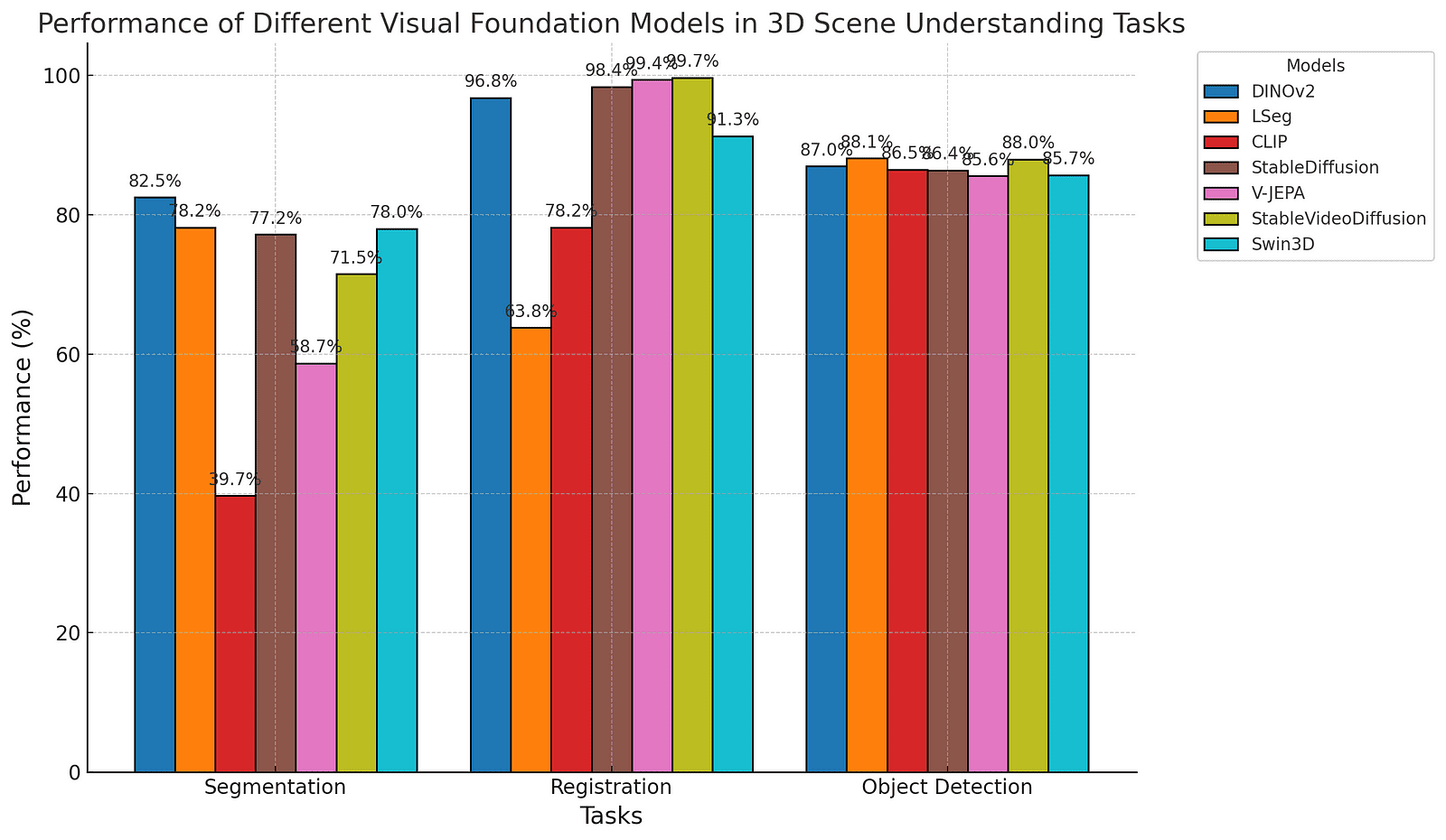
To help understand how different models perform in various 3D tasks, here is a graph above that shows the performance of different visual foundation models like DINOv2, Stable Video Diffusion, and others on tasks such as segmentation, registration, and object detection.
The Secret Behind DINOv2’s Superpowers
DINOv2, a self-supervised learning model, doesn’t rely on human-labeled data. Instead, it learns by observing patterns and making connections all on its own. This means it can adapt to new environments faster and with more flexibility than traditional models, making it incredibly useful for tasks like autonomous driving or robotics.
Video Models Have the Edge in Object-Level Tasks
Video models shine in tasks that involve understanding the movement of objects over time. Because they process continuous frames, they can tell the difference between similar-looking objects in a scene, like distinguishing between two identical chairs placed at different angles. This ability makes them invaluable for applications where distinguishing between similar items is crucial.
Why Diffusion Models Are Great at Geometric Tasks
Diffusion models like Stable Video Diffusion are particularly good at understanding the geometry of a scene. They excel in tasks that require aligning different viewpoints or piecing together partial images to form a complete picture, making them essential for applications like 3D modeling and VR content creation.
Language Models Aren’t Always the Best for Language Tasks
Surprisingly, models like CLIP, which are trained with language guidance, don’t always perform well on language-related tasks in 3D environments. This suggests that being good with words doesn’t necessarily make a model good at understanding complex visual scenes, challenging common assumptions in AI research.
3D Scene Understanding Could Revolutionize Search and Rescue
Imagine using AI to scan a disaster site, creating a 3D map of the rubble, and finding the safest path to trapped survivors. This is no longer just science fiction. With advanced 3D scene understanding, rescue operations could become faster, safer, and more effective, saving lives in the process.
The Future of Machine Vision
The evolution from flat, 2D image recognition to complex 3D scene understanding is more than just a technological leap. It’s a paradigm shift that will open up new possibilities for how we interact with the world. Imagine machines that truly understand the spaces we live in, that can navigate, explore, and solve problems in real time. This isn’t just about smarter technology; it’s about a future where the boundaries between digital and physical blur, where intelligent machines become our collaborators in creativity, exploration, and life-saving missions. The real world is three-dimensional, and now, finally, machines are catching up.
About Disruptive Concepts
https://disruptive-concepts.com/
Welcome to @Disruptive Concepts — your crystal ball into the future of technology. 🚀 Subscribe for new insight videos every Saturday!
Discover the Must-Have Kitchen Gadgets of 2024! From ZeroWater Filters to Glass Containers, Upgrade Your Home with Essential Tools for Safety and Sustainability. Click Here to Transform Your Kitchen Today!