Data surrounds us and binds us, silently collected and processed every day. From the moment you open an app, your data flows through systems, feeding statistics and trends. But who ensures your privacy when your information is shared? Differential privacy steps in as a mathematical guardian, adding noise to the data so that no one can tell if your record was there or not. It’s like hiding your voice in a crowd, keeping your identity safe while the crowd’s overall sound remains clear. This ensures that personal details never get exposed, even if someone’s trying to listen closely. As our world grows more connected, this invisible layer of protection becomes increasingly critical for governments and businesses alike.
Slowly Scaling Privacy: Protecting the Big and Small
Handling privacy becomes tricky when data sets grow large, especially when some data points are much more impactful than others. Think of income data where a single billionaire might skew the results. Traditional privacy methods struggle here. Slowly scaling privacy is a game-changer. It applies noise, or randomization, more effectively to highly influential records, ensuring their presence can’t be guessed without compromising the overall usefulness of the data. This breakthrough is especially important for releasing data on big entities, like major corporations or very wealthy individuals. By protecting even the biggest outliers, slowly scaling mechanisms keep everyone’s information equally safe.
The Algorithms That Protect Us
At the core of slowly scaling mechanisms is an impressive balancing act: protect privacy while keeping data accurate enough to be useful. Imagine you’re adding a layer of fog over a landscape. You want to blur the details just enough to hide specific points but not too much that you can’t recognize the shape of the hills. In technical terms, this means applying complex algorithms, like the transformation or additive mechanisms, to data. These tools help create a protective fog that gets denser for bigger, more influential records, all while maintaining a clear view of the overall data landscape. This technological breakthrough allows governments and businesses to release information safely, without the risk of revealing personal or sensitive details.
The Future of Trustworthy Data Sharing
Differential privacy is already being used in critical places, like the U.S. Census. But with slowly scaling privacy, we’re taking it to the next level. It opens the door for safer, more widespread sharing of data, especially in fields like economics, healthcare, and technology. Companies can release more accurate reports without risking individual privacy, and governments can offer detailed public statistics while ensuring citizens are protected. It’s not just about privacy; it’s about trust. By implementing these mechanisms, we are creating a future where data can fuel progress without ever compromising personal security.
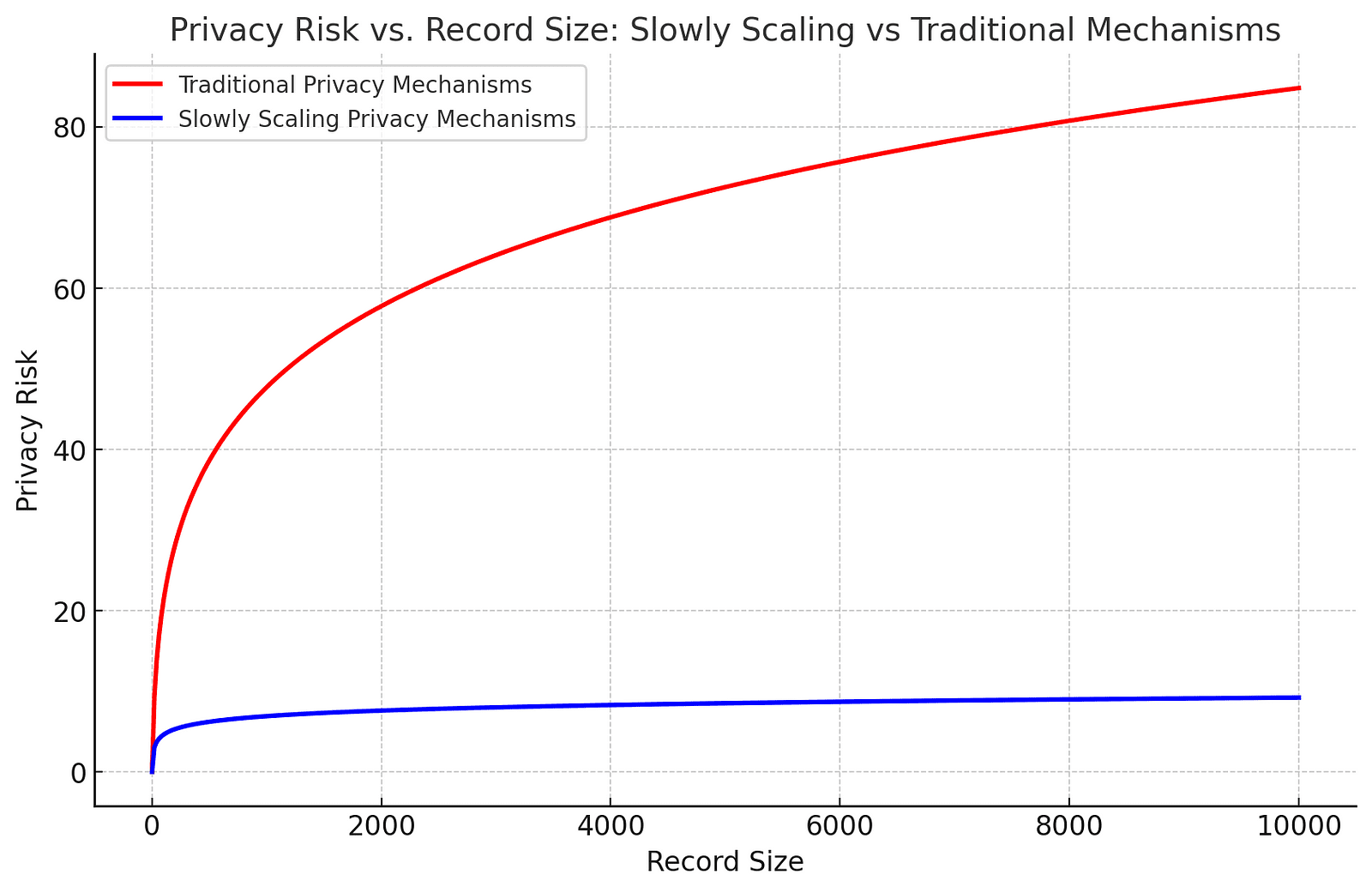
The graph above shows how privacy risk changes as the record size increases, comparing traditional privacy mechanisms with slowly scaling mechanisms. As demonstrated, traditional methods see a sharp rise in privacy risk with larger records, while slowly scaling mechanisms maintain better protection, keeping the risk significantly lower, even for influential records.
Bigger Isn’t Safer
Traditional privacy methods can struggle to protect outliers, like the wealthiest individuals or biggest corporations in a data set. Slowly scaling mechanisms solve this by adapting the privacy protection for these influential records.
Less Noise, More Accuracy
Slowly scaling privacy mechanisms allow for less noise to be added to most data points, improving the overall accuracy of released statistics while maintaining privacy.
Used in National Statistics
The U.S. Census has already adopted differential privacy techniques, showing how critical this technology is in safeguarding large-scale public data sets.
Protecting the Unseen
This privacy mechanism can hide the presence of any individual record so well that even the most detailed statistical analysis cannot reveal if someone’s data was included.
Broad Applications Beyond Economics
While slowly scaling privacy is excellent for economic data, it’s also vital for fields like healthcare, where certain medical records can heavily influence data trends.
The Bright Future of Privacy-Protected Data
As we move further into the digital age, privacy protection becomes more than just an option — it’s a necessity. Slowly scaling differential privacy gives us a glimpse into a future where data sharing can happen on a massive scale, without the fear of compromising personal details. This technology ensures that no matter how large or small your contribution is, your privacy remains intact. It’s a bold step towards a safer, more transparent world where data can drive progress without putting anyone at risk.
About Disruptive Concepts
Welcome to @Disruptive Concepts — your crystal ball into the future of technology. 🚀 Subscribe for new insight videos every Saturday!
See us on https://twitter.com/DisruptConcept
Read us on https://medium.com/@disruptiveconcepts
Enjoy us at https://www.disruptive-concepts.com
Whitepapers for you at: https://disruptiveconcepts.gumroad.com/l/emjml